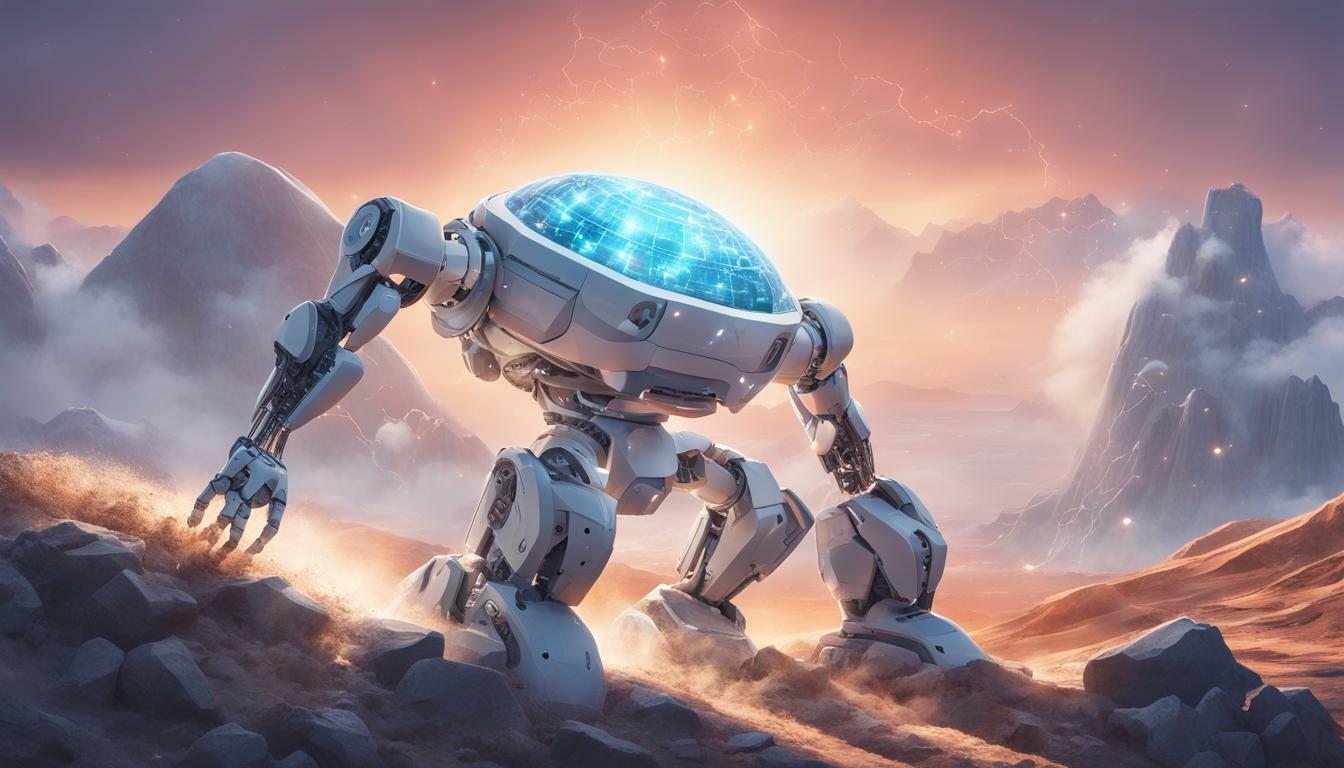
The development of artificial intelligence (AI) has opened up new possibilities and opportunities across various industries. However, AI development is not without its challenges. Two significant challenges are bias in data and lack of transparency in AI systems. Addressing these challenges is crucial for the responsible development and deployment of AI systems.
Key Takeaways:
- Challenges in AI development include data bias and transparency issues.
- Addressing these challenges is necessary for responsible AI development and deployment.
Understanding Data Bias in AI
Artificial Intelligence (AI) has become a significant driving force in shaping the future of various industries. Despite its potential benefits, AI development poses several challenges that require attention. One significant challenge is data bias, which can significantly impact the accuracy and fairness of AI systems.
Data bias occurs when the data used to train AI algorithms is unrepresentative or contains systematic errors that produce biased results. This issue arises due to various factors, including biased data collection methods or pre-existing societal biases that reflect in the data used to train AI algorithms.
Addressing data bias in AI is crucial because biased AI systems can lead to adverse outcomes in decision-making, affect the well-being of underrepresented groups and undermine public trust in AI. The development of fair and unbiased AI systems is therefore essential for their responsible and ethical deployment.
Challenges in Addressing Data Bias in AI
Addressing data bias in AI development is a complex task that poses several challenges. One of the primary difficulties is the lack of diverse representation in training data. This issue impacts the accuracy of AI algorithms, as they may make incorrect assumptions or predictions based on limited or biased data. Another significant challenge is the difficulty in identifying and mitigating biases in AI systems, particularly when the bias is implicit and difficult to identify.
Moreover, solving data bias in AI development requires interdisciplinary collaboration between technical and non-technical experts. Such collaborations are necessary to ensure that all perspectives are considered and that the resulting AI systems are inclusive and representative of diverse groups.
Strategies to Address Data Bias in AI
There are various strategies and approaches that can be used to address data bias in AI development. One common technique is data preprocessing, where training data is preprocessed to remove any biases that may exist. Another approach is algorithmic fairness, which involves adjusting the algorithm used to ensure that the output is fair and unbiased.
In addition, ensuring diverse representation in training data can mitigate data bias in AI. This can be achieved by collecting data from a diverse range of sources, including underrepresented groups. Ongoing monitoring and evaluation of AI systems can also identify any biases that may arise and enable them to be addressed in a timely manner.
The development of standard frameworks and guidelines for the ethical and responsible deployment of AI systems can also mitigate data bias in AI. Such frameworks can outline the best practices for data collection, model development, and deployment of AI systems, thus promoting fairness, transparency, and accountability.
Addressing data bias in AI development is a crucial step in ensuring that AI systems are trustworthy, inclusive, and represent the diversity of the communities they serve. By implementing strategies and approaches that address data bias, AI systems can become more accurate, fair, and unbiased.
Ensuring Transparency in AI Development
Transparency is essential in developing trustworthy AI systems. It ensures that AI systems are reliable, safe, and do not perpetuate bias or discrimination. The lack of transparency in AI systems is one of the most significant challenges in artificial intelligence development, hindering its adoption and acceptance.
To enable transparency in AI systems, developers must consider several aspects. Firstly, it is crucial to ensure explainability, which means that the AI system’s decisions and predictions must be understandable and interpretable by humans. Secondly, developers must ensure accountability, which requires clear documentation of the AI system’s design, development, and decision-making processes. Finally, auditability is crucial to ensure transparency. Developers must ensure that the AI system’s decisions and output are traceable and recorded for review and evaluation.
One of the significant challenges in AI transparency is the lack of standardization of transparency requirements. Different stakeholders have varying expectations and interpretations of what constitutes transparency in AI systems, making it challenging to develop universally accepted standards. However, initiatives such as the IEEE Global Initiative for Ethical Considerations in Artificial Intelligence and Autonomous Systems and the EU’s General Data Protection Regulation are pushing for transparency and accountability in AI systems.
Ensuring transparency is crucial in building trust and acceptance of AI systems. Transparent and accountable AI systems will increase their adoption in various industries, benefiting society as a whole. Addressing transparency issues in AI development requires an open, collaborative effort from all stakeholders, including developers, researchers, industry leaders, policymakers, and end-users.
Ethical Implications of Data Bias and Lack of Transparency
The challenges related to data bias and transparency issues in AI systems have significant ethical implications. Data bias can lead to discriminatory decisions, perpetuating social and economic inequality. For instance, algorithms that are trained on biased data can result in biased hiring or lending decisions. Similarly, a lack of transparency makes it difficult to identify and address such biases. It can also erode trust in AI systems, hindering their adoption and potential benefits.
To ensure that AI systems are developed and deployed responsibly, it is essential to address these challenges. Ethical frameworks and guidelines can help to promote fairness and inclusivity. They can also provide a basis for the development of regulatory standards that promote transparency in AI systems.
The impact of data bias and lack of transparency is not just limited to technical issues. It has broader societal implications. AI systems are increasingly being used in decision-making processes that affect people’s lives. It is essential that these systems be transparent, explainable, and accountable to ensure that they do not perpetuate discrimination or harm.
As such, addressing data bias and transparency issues in AI development is critical in ensuring that AI systems are developed and deployed responsibly. It requires ongoing collaboration between researchers, industry leaders, policymakers, and society at large.
Strategies to Address Data Bias in AI
Data bias is a significant challenge in AI development and can lead to unfair or inaccurate outcomes. Here are some strategies to address data bias in AI:
Data Preprocessing
Data preprocessing involves identifying and correcting biases in the training data used to build AI models. To do this, it’s important to analyze the data for any imbalances, such as overrepresentation or underrepresentation of certain groups. Once identified, the biased data can be removed or adjusted to ensure a more diverse and representative dataset.
Algorithmic Fairness
Algorithmic fairness aims to ensure that AI systems are designed to produce equitable results. To achieve this, it’s essential to identify and eliminate any bias that may exist in the algorithms themselves. The approach involves creating algorithms that are specifically designed to eliminate or counteract any potential biases in the data.
Diverse Representation in Training Data
AI systems need to be trained on diverse and representative datasets. This means ensuring that the data used to train the models reflects the diversity of the users who will be using them. Diverse representation in training datasets can help to reduce the impact of any biases that may exist and lead to more accurate and fair outcomes.
By addressing data bias in AI through these strategies and ongoing monitoring and evaluation, we can create more fair and unbiased AI systems that benefit everyone.
Enhancing Transparency in AI Development
Transparency is a key challenge in AI development, primarily due to the complexity of AI systems, the lack of insight into the decision-making processes, and the inherent opacity of many machine learning algorithms. Ensuring transparency in AI development is vital to building trust and acceptance among users and stakeholders, and promoting the responsible and ethical deployment of AI systems.
One of the primary aspects of transparency in AI development is explainability, which refers to the ability to understand and interpret the decisions made by AI systems. This requires clear documentation of AI models and algorithms, as well as the ability to audit and analyze the data inputs and outputs of AI systems.
Algorithmic accountability is another important aspect of transparency, which refers to the ability to track and monitor the performance of AI systems over time. This requires ongoing evaluation and testing of AI models, as well as the establishment of clear standards and guidelines for responsible AI development and deployment.
The role of regulatory frameworks and standards is another important factor in enhancing transparency in AI development. These frameworks and standards can help ensure that AI systems are developed and deployed in a responsible and ethical manner, and provide a clear framework for evaluating and addressing potential issues related to data bias and transparency issues.
Ultimately, enhancing transparency in AI development requires a concerted effort from all stakeholders, including industry leaders, policymakers, and researchers. By working together to address the challenges faced in implementing transparent and ethical AI systems, we can build a future where AI is used to enhance human capabilities and improve our lives.
Collaborative Efforts and Industry Initiatives
The challenges in AI development, ranging from data bias to transparency issues, require collective efforts from industry players, policymakers, and researchers. Initiatives are being taken on multiple fronts to address these challenges.
One of the notable initiatives is the Partnership on AI, which is a non-profit organization aimed at ensuring AI’s responsible development and deployment. Its members include major tech companies such as Google, Facebook, and IBM, along with NGOs and academic institutions.
Another initiative is the AI Now Institute, which is a research institute focused on studying the social implications of AI. It produces annual reports highlighting the latest research on AI policy, bias, and accountability.
Additionally, regulatory bodies are taking action to promote responsible AI development. For instance, the European Union’s General Data Protection Regulation (GDPR) has provisions for protecting individual privacy rights, which also apply to AI systems. Similarly, the Algorithmic Accountability Act and the Ethical AI Act are two bills introduced in the US Congress to require companies to assess and address bias in their AI systems.
Collaborations between industry and academia are also fostering research and development on ethical AI systems. The Fairness, Accountability, and Transparency in Machine Learning (FAT/ML) community brings together researchers and practitioners to discuss challenges related to fairness and accountability in ML, while the AI Ethics Lab is a research institute focused on AI ethics and policy.
In summary, initiatives and collaborations on multiple fronts are working towards mitigating the challenges in AI development. By working together, industry players, policymakers, and researchers can pave the way for the responsible and ethical deployment of AI systems.
Conclusion
In conclusion, addressing challenges related to data bias and transparency is crucial in the responsible and ethical deployment of AI systems. Eliminating data bias in AI requires ongoing monitoring and evaluation, diverse representation in training data, and algorithmic fairness. Enhancing transparency in AI development involves clear documentation, auditability, and algorithmic accountability, as well as regulatory frameworks and standards.
As AI becomes increasingly integrated into various industries, it is essential to prioritize transparency and fairness. Failure to address these challenges may result in bias in decision-making processes, with potential ethical implications and negative impacts on inclusivity and diversity. Collaborative efforts among researchers, industry leaders, and policymakers are crucial in overcoming these challenges and ensuring the development of responsible and ethical AI systems.
Call to Action
It is essential for the industry to work collectively towards overcoming these challenges and prioritizing transparency and fairness in AI development. By working together, we can ensure the responsible deployment of AI systems that benefit society as a whole.
FAQ
Q: What are the challenges in AI development?
A: The challenges in AI development include data bias and transparency issues.
Q: What is data bias in AI?
A: Data bias in AI refers to the skewed representation of certain groups or characteristics in the training data, leading to biased outcomes and decisions.
Q: How does data bias impact AI systems?
A: Data bias can result in discriminatory outcomes and reinforce societal biases, affecting fairness and inclusivity in AI systems.
Q: Why is transparency important in AI development?
A: Transparency is crucial in AI development to build trust, understand decision-making processes, and ensure accountability.
Q: What aspects need to be addressed to ensure transparency in AI?
A: Aspects such as explainability, interpretability, clear documentation, and algorithmic accountability need to be addressed to ensure transparency in AI.
Q: What are the ethical implications of data bias and lack of transparency in AI?
A: Data bias and lack of transparency in AI can lead to unfair and discriminatory outcomes, impacting ethical considerations such as fairness and inclusivity.
Q: What strategies can be used to address data bias in AI?
A: Strategies to address data bias in AI include data preprocessing, algorithmic fairness, and diverse representation in training data.
Q: How can transparency be enhanced in AI development?
A: Transparency in AI development can be enhanced through clear documentation, auditability, and regulatory frameworks promoting algorithmic accountability.
Q: What collaborative efforts and industry initiatives exist to address AI development challenges?
A: Researchers, industry leaders, and policymakers are involved in collaborative efforts and initiatives aimed at mitigating data bias and enhancing transparency in AI systems.
Q: What is the importance of addressing challenges in AI development?
A: Addressing challenges in AI development, such as data bias and transparency issues, is essential for the responsible and ethical deployment of AI systems.